In-Distribution vs. Out-of-Distribution Ideas
Why ideas matter and how AI models allow us to litigate novelty, creativity, complexity, and depth of ideas and life
A simple definition from Claude for the uninitiated: In essence, in-distribution data fits the patterns the AI is trained on, while out-of-distribution data presents new, unfamiliar patterns or scenarios.
—
The human condition is romantic. We tightly guard and appreciate the idea that we are all unique, each with our own novelty running through our veins and shooting out of each electrical charge from our neurons firing. We as humans are connected because we have both thought what others have thought and only think what no others have ever thought.
The concept of some form of heightened superintelligence threatens this. LLMs broadly take the collective thoughts of humanity and shine a mirror on those writing prompts, spitting out an answer that could lead to a given person learning that perhaps so many others have thought everything we have thought. And we will likely learn, that that's okay in some regards.
AI as a Novelty Barometer
One of the most surprisingly delightful experiences about using LLMs for me has been the ability for them to litigate novelty. While we're all eagerly awaiting LLMs to produce truly emergent behaviors or ideas beyond their training data, I think this expectation might be misplaced. What we're really asking is: can a simple creative prompt lead an LLM to generate genuinely novel research, discoveries, or ideas?
Until that happens, I think LLMs are good mirrors for how novel your ideas are, and they help you litigate whether you as a person/group of people should be having more in-distribution (familiar) or out-of-distribution (novel) ideas.
Put another way, if through simple prompting an LLM reveals an idea/thought with high degree of similarity to your own, it's a good proxy that you may need to think more creatively (find ideas the LLM can't come to simply) or deeply (navigate the depth and nuance of ideas the LLM won't come to).
Ideas can come in forms of specific and abstract visions. A way to litigate the degree of abstract topic novelty is use a tool like WebSim to see just how in distribution your ideas look without material prompting. Doing so allows you to feed in an abstract vision and let the model interpret it for you to see if you still come back as merely an output within a standard deviation of training data.
For example, I put in minimal information about Compound to WebSim, asked for a future portfolio page from the year 2030, and got back a list of companies with some of our private theses (and in some cases even still stealth investments) in it. This shows these ideas aren’t that novel.
In-distribution ideas are not always bad.
Sometimes the best versions of in-distribution ideas arise because of human error. As people continue to aggressively sort into non-gradients and only seek reinforcement in their opinions, they can become more close-minded to re-visiting topics or to shifting their beliefs for a variety of reasons, despite the fact that the world and feelings are constantly evolving.
As models are effectively constantly trained and thus continually update with new information1, they (theoretically) don't have these built-in biases in the same ways that humans do, which means they can help you reason about heavily-biased topics easily.
I find that that is where the in-distribution ideas are special; when one can re-litigate past ideas that have been cast in stone or discarded into a permanent place of irrelevancy or nothingness.
Moving Out of Distribution
Out-of-distribution ideas feel unique.
They are the result of us stepping into a realm where our ability to draw from diverse experiences and make intuitive leaps gives us humans a unique advantage or novelty relative to the weights of a model.
Some out-of-distribution ideas successfully create something intersectional or translational between multiple areas. It's in this cognitive fog of war that we start to push on creativity and novelty.
There's a concept called technological convergence that happens effectively when two different types of technologies are brought together in order to form a new technology2. This framing of recognizing the interplay of two well-known technologies to arrive at a novel technology bares a lot of similarity to a version of out-of-distribution ideas.
Life often happens via a series of seemingly random chain-reaction events and sliding door moments. The beauty of strings of randomness means that you have an immense amount of error rates that can compound over time across a series of events/interactions, which perhaps speaks to why connections amongst people are so special. So many things theoretically have to go right.
Out of distribution ideas can be those that require multi-step long-term reasoning or complex navigation of gradients of possibilities. This level of complexity has thus far largely evaded models, though there are hypotheses that scaling inference time will lead to better ability to navigate proverbial idea mazes (OpenAI’s o1 models are the first signals of this to some degree).
These types of out-of-distribution ideas can take on many different forms, from navigating broader themes around forecasting all the way to finding the resulting "abstractions" that actually get to the core of what a long term vision is.
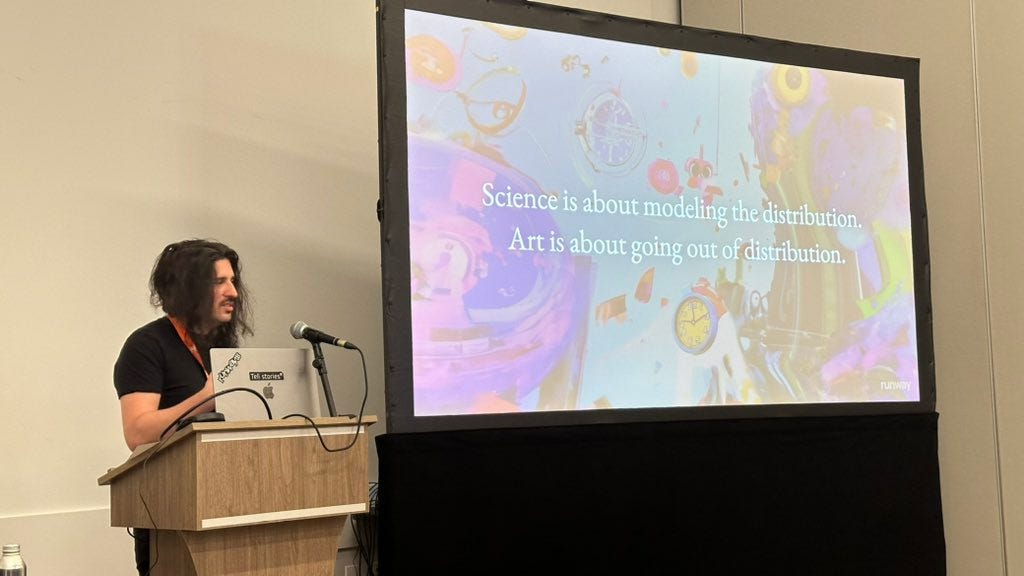
On the latter, I'm often reminded of Runway’s take, when they say they aren't building video generation models or world models, but instead they're building a new camera and the next era of art.
While to some this, along with "growing the GDP of the internet" (Stripe) and "making life multi-planetary" (SpaceX) sounds unnecessarily abstract or grandiose at first, these framings create baseline understanding and convey the prescience of what the long-term mission is of special long-term oriented ideas and importantly, frame the heart of the idea that sits in a gray area.3 An almost precise abstraction, you could say.
These abstractions and out-of-distribution ideas aren't just intellectual exercises, they're the guideposts for creating a form of truly transformative work.
As AI gets better at churning out obvious ideas, our human advantage and comparative advantages lie in our ability to generate and build upon novel concepts. This is why developing out-of-distribution thinking is so crucial. It's not about asking AI to list every possible solution (though I’m sure someone will say this is the endgame of superintelligence); it's about venturing into areas where AI's current (and maybe future) capabilities fall short.
The real value emerges from connecting these novel ideas and/or forming new patterns of thought that AI struggles to replicate on top of in-distribution ideas. This approach pushes us to think beyond immediate limitations or incrementalism and instead consider possibilities that might seem far-fetched at first. It reminds us that while AI can help navigate the familiar, exploring the unknown in some ways may remain uniquely human. In a world where the obvious is increasingly automated and discoverable, our ability to work in these gray areas becomes not just valuable, but essential.
And life mostly happens in gray areas.
Let’s not get hung up on the cadence of pre-training etc. A new model comes out every 12 months at most and has updated context.
I wrote about this more in-depth in On Inflection Points
To be fair, there is some survivorship bias here. A lot of companies make these grandiose type statements in an effort of abstract the actual lack of creativity or to overcomplicate and stretch the company narrative.